ERAU Industrial Math Project: Characterizing Seismic Events through Advanced Data Analytics
PI Mihhail Berezovski
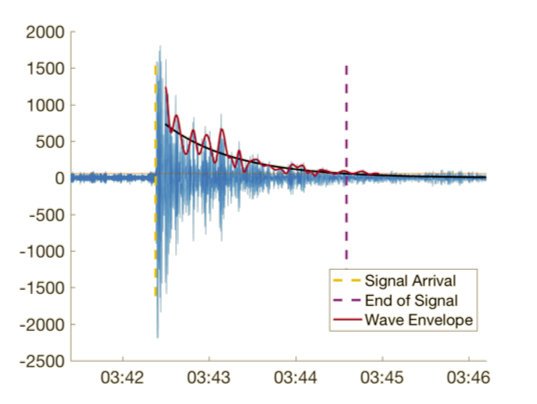
The Signal Processing and Applied Mathematics research group at the Nevada National Security Site, is seeking to collaborate with students at Embry-Riddle Aeronautical University to develop advanced data analytics methods for characterizing seismic events in the southwest United States as near-field earthquakes, far-field earthquakes, or non-natural seismic events, using publicly available seismic data.
One way of monitoring whether other countries are engaging in explosives-driven testing of weapons is to measure seismicity from the events, but this is only interesting if we can correctly characterize a seismic event as an:
- earthquake,
- industrial explosives event (mining, construction, etc.),
- or an explosives test.
The first step is to simply be able to distinguish between natural and non-natural seismic events. Since the Nevada National Security Site engages in explosives-driven tests - and is in an area of active earthquake activity - it is a natural test bed for developing data analytics approaches to classifying signals as natural or non-natural. Being a "test bed" means that, in addition to the measured signals, we have some ground truth information, meaning we know when earthquakes and explosives tests actually happen.
Larger-scale experiments, like the North Korean nuclear tests, can be seen at much larger distances, so even in Nevada we can measure seismic signals from nuclear tests. Figure shows a seismic measurement in Nevada from North Korea's September 2017 nuclear test.
Primary Goal: Develop analytics methods for differentiating near- field, small earthquakes in Nevada and southern California, from far- field, large earthquakes around the world, from non-natural seismic events.
One of the primary challenges in analyzing seismic data is that the data is collected 24 hours per day, 7 days a week, but actual seismic anomalies - either natural or not - occur relatively rarely.
Thus a first step in the analytics is to develop a technique for combing through the data to find anything that looks like an anomaly. The second task will be to classify the anomalies.
Research Dates
01/09/2019 to 01/09/2021